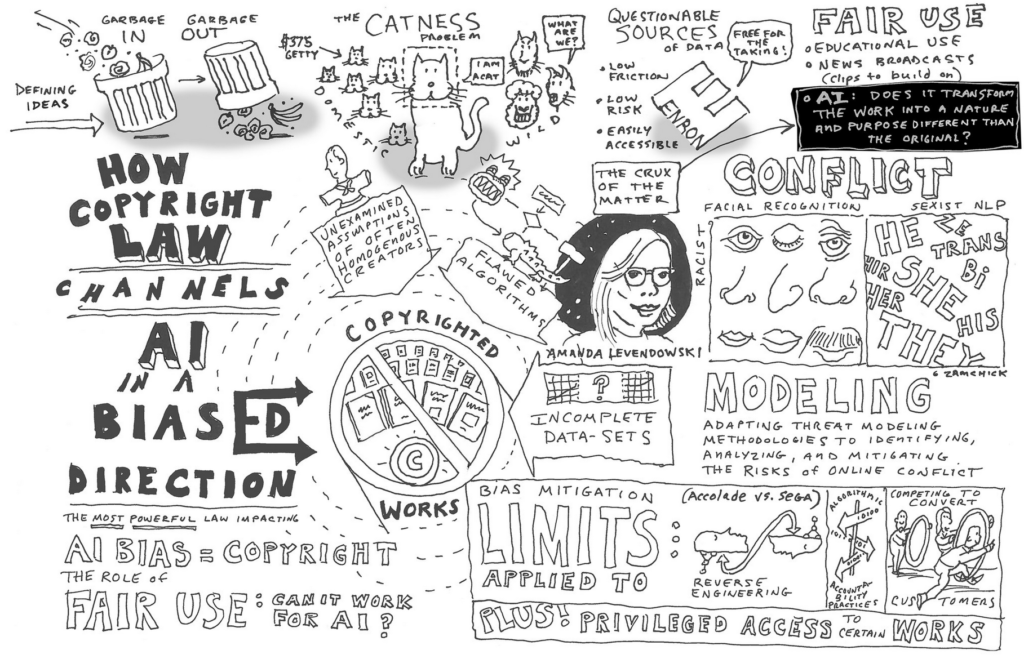
Illustration courtesy of Gary Zamchick | Used with permission
The principles of copyright law sometimes have a way of appearing in unexpected places. Recently we featured an article by Christopher Sprigman that examines assumptions about copyright as a spur to creativity by considering examples as diverse as Italian opera and Bollywood movies.
Today, as part of Fair Use Week, we are highlighting new research by NYU Clinical Teaching Fellow Amanda Levendowski that explores the ways in which copyright law can negatively influence the quality of artificial intelligence (AI), and how fair use might be part of the solution. She describes how there has been an increase in examples of AI systems reflecting or exacerbating societal bias, from racist facial recognition to sexist natural language processing.
As the computer science adage “garbage in, garbage out,” succinctly puts it, an AI system is only as good as the information provided to it. Training using biased or otherwise unsatisfactory data can result in flawed and incomplete outcomes. As Levendowski writes, “[J]ust as code and culture play significant roles in how AI agents learn about and act in the world, so too do the laws that govern them. … The rules of copyright law…privilege access to certain works over others, encouraging AI creators to use easily available, legally low-risk sources of data for teaching AI, even when those data are demonstrably biased.”
With potential statutory damages running as high $150,000 per infringed work, AI creators often to turn to easily available, legally low-risk works train AI systems, often resulting in what Levendowski calls “biased, low-friction data” (BLFD). One such example is the use of the “Enron emails”, the 1.6 million emails sent among Enron employees that are publicly available online, as a go-to dataset for training AI systems. As Levendowski puts it, “If you think that there might be significant biases embedded in emails sent among employees of [a] Texas oil-and-gas company that collapsed under federal investigation for fraud stemming from systemic, institutionalized unethical culture, you’d be right: researchers have used the Enron emails specifically to analyze gender bias and power dynamics.”
What’s more, Levendowski describes how copyright law favors incumbent AI creators who can use training data that are a byproduct of another activity (such as the messages and photos Facebook uses to train its systems) or that it can afford to purchase. This can play a determinative role in which companies can effectively compete in the marketplace.
So how can we fix AI’s implicit bias problem? In her article, Levendowski argues that if we hope to create less biased AI systems, we need to use copyrighted works as training data. Happily, copyright law has built-in tools that help to balance the interests of copyright owners against the interests of onward users and the public: One of these tools is fair use. By examining the use of copyrighted works as AI training data through the lens of fair use cases involving computational technologies, Levendowski suggests that relying on fair use to use copyrighted materials in training systems could provide a promising path forward to combat bias and make AI more inclusive and more accurate.
Read the full article on SSRN; and learn more about Levendowski and her research on her website.
Amanda Levendowski is a Clinical Teaching Fellow with the NYU Technology Law and Policy Clinic. Her clinical projects and research address how we can develop practical approaches to digital problems. Amanda previously practiced copyright, trademark, Internet, and privacy law at Kirkland & Ellis and Cooley LLP.