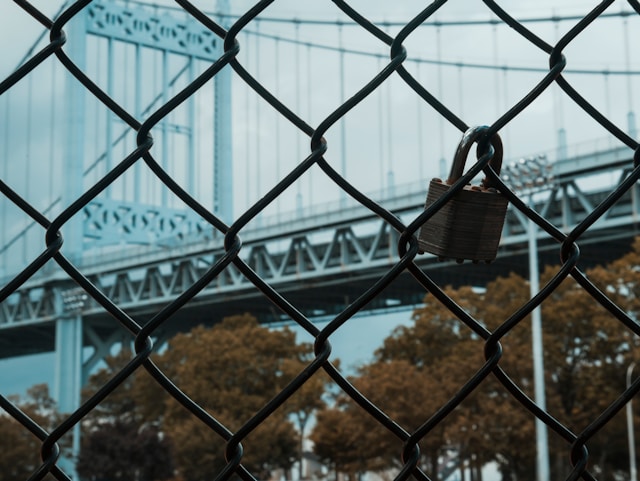
This post is by Rachael Samberg, Director, Scholarly Communication & Information Policy, UC Berkeley Library and Dave Hansen, Executive Director, Authors Alliance
This post is about the research and the advancement of science and knowledge made impossible when publishers use contracts to limit researchers’ ability to use AI tools with scholarly works.
Within the scholarly publishing community, mixed messages pervade about who gets to say when and how AI tools can be used for research reliant on scholarly works like journal articles or books. Some scholars voiced concern (explained more here) when major scholarly publishers like Wiley or Taylor & Francis entered lucrative contracts with big technology companies to allow for AI training without first seeking permission from authors. We suspect that these publishers have the legal right to do so since most publishers demand that authors hand over extensive rights in exchange for publishing their work. And with the backdrop of dozens of pending AI copyright lawsuits, who can blame the AI companies for paying for licenses, if for no other reason than avoiding the pain of litigation? While it stings to see the same large commercial, academic publishers profit yet again off of the work academic authors submit to them for free, we continue to think there are good ways for authors to retain a say in the matter.
Big tech companies are one thing, but what about scholarly research? What about the large and growing number of scholars who are themselves using scholarly copyrighted content with AI tools to conduct their research? We currently face a situation in which publishers are attempting to dictate how and when researchers can do that work, even when authors’ fair use rights to use and derive new understandings from scholarship clearly allow for such uses.
How vendor contracts disadvantage US researchers
We have written elsewhere (in an explainer and public comment to the Copyright Office) why training AI tools, particularly in the scholarly and research context, constitutes a fair use under U.S. Copyright law. Critical for the advancement of knowledge, training AI is based on a statutory right already held by all scholarly authors engaging in computational research and one that lawmakers should preserve.
The problem U.S. scholarly authors presently face with AI training is that publishers restrict their access to these statutory rights through contracts that override them: In the United States, publishers can use private contracts to take away statutory fair use rights that researchers would otherwise hold under Federal law. In this case, the private contracts at issue are the electronic resource (e-resource) license agreements that academic research libraries sign to secure campus access to electronic journal, e-book, data, and other content that scholars need for their computational research.
Contractual override of fair use is a problem that disparately disadvantages U.S. researchers. As we have described elsewhere, more than forty countries, including the European Union, expressly reserve text mining and AI training rights for scientific research by research institutions. Not only do scholars in these countries not have to worry whether their computational research with AI is permitted, but also: They do not risk having those reserved rights overridden by contract. The European Union’s Copyright Digital Single Market Directive and recent AI Act nullify any attempt to circumscribe the text and data mining and AI training rights reserved for scientific research within research organizations. U.S. scholars are not as fortunate.
In the U.S., most institutional e-resource licenses are negotiated and managed by research libraries, so it is imperative that scholars work closely with their libraries and advocate to preserve their computational research and AI training rights within the e-resource license agreements that universities sign. To that end, we have developed adaptable licensing language to support institutions in doing that nationwide. But while this language is helpful, the onus of advocacy and negotiation for those rights in the contracting process remains. Personally, we have found it helpful to explain to publishers that they must consent to these terms in the European Union, and can do so in the U.S. as well. That, combined with strong faculty and administrative support (such as at the University of California), makes for a strong stance against curtailment of these rights.
But we think there are additional practical ways for libraries to illustrate—both to publishers and scholarly authors—exactly what would happen to the advancement of knowledge if publishers’ licensing efforts to curtail AI training were successful. One way to do that is by “unpacking” or decoding a publisher’s proposed licensing restriction, and then demonstrating the impact that provision would have on research projects that were never objectionable to publishers before, and should not be now. We’ll take that approach below.
Decoding a publisher restriction
A commercial publisher recently proposed the following clause in an e-resource agreement:
Customer [the university] and its Authorized Users [the scholars] may not:
- directly or indirectly develop, train, program, improve, and/or enrich any artificial intelligence tool (“AI Tool”) accessible to anyone other than Customer and its Authorized Users, whether developed internally or provided by a third party; or
- reproduce or redistribute the Content to any third-party AI Tool, except to the extent limited portions of the Content are used solely for research and academic purposes (including to train an algorithm) and where the third-party AI Tool (a) is used locally in a self-hosted environment or closed hosted environment solely for use by Customer or Authorized Users; (b) is not trained or fine-tuned using the Content or any part thereof; and (c) does not share the Content or any part thereof with a third party.
What does this mean?
- The first paragraph forbids the training or improving of any AI tool if it’s accessible or released to third parties. And, it further forbids the use of any computational outputs or analysis that are derived from the licensed content from being used to train any tool available to third parties.
- The second paragraph is perhaps even more concerning. It provides that when using third party AI tools of any kind, a scholar can use only limited portions of the licensed content with the tools, and are prohibited from doing any training at all of third party tools even if it’s a non-generative AI tool and the scholar is performing the work in a completely closed and highly secure research environment.
What would the impact of such a restrictive licensing provision be on research?
It would mean that every single one of the trained tools in the following projects could never be disseminated. In addition, for the projects below that used third-party AI tools, the research would have been prohibited full-stop because the third-party tools in those projects required training which the publisher above is attempting to prevent:
Tools that could not be disseminated
- In 2017, chemists created and trained a generative AI tool on 12,000 published research papers regarding synthesis conditions for metal oxides, so that the tool could identify anticipated chemical outputs and reactions for any given set of synthesis conditions entered into the tool. The generative tool they created is not capable of reproducing or redistributing any licensed content from the papers; it has merely learned conditions and outcomes and can predict chemical reactions based on those conditions and outcomes. And this beneficial tool would be prohibited from dissemination under the publisher’s terms identified above.
- In 2018, researchers trained an AI tool (that they had originally created in 2014) to understand whether a character is “masculine” or “feminine” by looking at the tacit assumptions expressed in words associated with that character. That tool can then look at other texts and identify masculine or feminine characters based on what it knows from having been trained before. The implications are that scholars can therefore use texts from different time periods with the tool to study representations of masculinity and femininity over time. No licensed content, no licensed or copyrighted books from a publisher can ever be released to the world by sharing the trained tool; the trained tool is merely capable of topic modeling—but the publisher’s above language would prohibit its dissemination nevertheless.
Tools that could neither be trained nor disseminated
- In 2019, authors used text from millions of books published over 100 years to analyze cultural meaning. They did this by training third-party non-generative AI word-embedding models called Word2Vec and GLoVE on multiple textual archives. The tools cannot reproduce content: when shown new text, they merely represent words as numbers, or vectors, to evaluate or predict how similar words in a given space are semantically or linguistically. The similarity of words can reveal cultural shifts in understanding of socioeconomic factors like class over time. But the publisher’s above licensing terms would prohibit the training of the tools to begin with, much less the sharing of them to support further or different inquiry.
- In 2023, scholars trained a third-party-created open-source natural language processing (NLP) tool called Chemical Data Extractor (CDE). Among other things, CDE can be used to extract chemical information and properties identified in scholarly papers. In this case, the scholars wanted to teach CDE to parse a specific type of chemical information: metal-organic frameworks, or MoFs. Generally speaking, the CDE tool works by breaking sentences into “tokens” like parts of speech and referenced chemicals. By correlating tokens, one can determine that a particular chemical compound has certain synthetic properties, topologies, reactions with solvents, etc. The scholars trained CDE specifically to parse MoF names, synthesis methods, inorganic precursors, and more—and then exported the results into an open source database that identifies the MoF properties for each compound. Anyone can now use both the trained CDE tool and the database of MoF properties to ask different chemical property questions or identify additional MoF production pathways—thereby improving materials science for all. Neither the CDE tool nor the MoF database reproduces or contains the underlying scholarly papers that the tool learned from. Yet, neither the training of this third-party CDE tool nor its dissemination would be permitted under the publisher’s restrictive licensing language cited above.
Indeed, there are hundreds of AI tools that scholars have trained and disseminated—tools that do not reproduce licensed content—and that scholars have created or fine-tuned to extract chemical information, recognize faces, decode conversations, infer character types, and so much more. Restrictive licensing language like that shown above suppresses research inquiries and societal benefits that these tools make possible. It may also disproportionately affect the advancement of knowledge in or about developing countries, which may lack the resources to secure licenses or be forced to rely on open-source or poorly-coded public data—hindering journalism, language translation, and language preservation.
Protecting access to facts
Why are some publishers doing this? Perhaps to reserve the opportunity to develop and license their own scholarship-trained AI tools, which they could then license at additional cost back to research institutions. We could speculate about motivations, but the upshot is that publishers have been pushing hard to foreclose scholars from training and dissemination AI tools that now “know” something based on the licensed content. That is, such publishers wish to prevent tools from learning facts about the licensed content.
However, this is precisely the purpose of licensing content. When institutions license content for their scholars to read, they are doing so for the scholars to learn information from the content. When scholars write about it or teach about the content, they are not regenerating the actual expression from the content—the part that is protected by copyright; rather the scholars are conveying the lessons learned from the content—facts not protected by copyright. Prohibiting the training of AI tools and the dissemination of those tools is functionally equivalent to prohibiting scholars from learning anything about the content that institutions are licensing for that very purpose, and that scholars have written to begin with! Publishers should not be able to monopolize the dissemination of information learned from scholarly content, and especially when that information is used non-commercially.
For these reasons, when we negotiate to preserve AI usage and training rights, we generally try to achieve the following outcomes which would promote—rather than prohibit—all of the research projects described above:

The sample language we’ve disseminated empowers others to negotiate for these outcomes. We hope that, when coupled with the advocacy tools we’ve provided above, scholars and libraries can protect their AI usage and training rights, while also being equipped to consider how they want their own works to be used.